In today’s rapidly evolving financial landscape, credit analysis automation has become a cornerstone for institutions striving to achieve efficiency and accuracy. Financial institutions worldwide are leveraging cutting-edge technology to revolutionize traditional credit processes, improving decision-making and operational speed. This transformative shift addresses growing challenges and ensures sustainable growth in a highly competitive market.
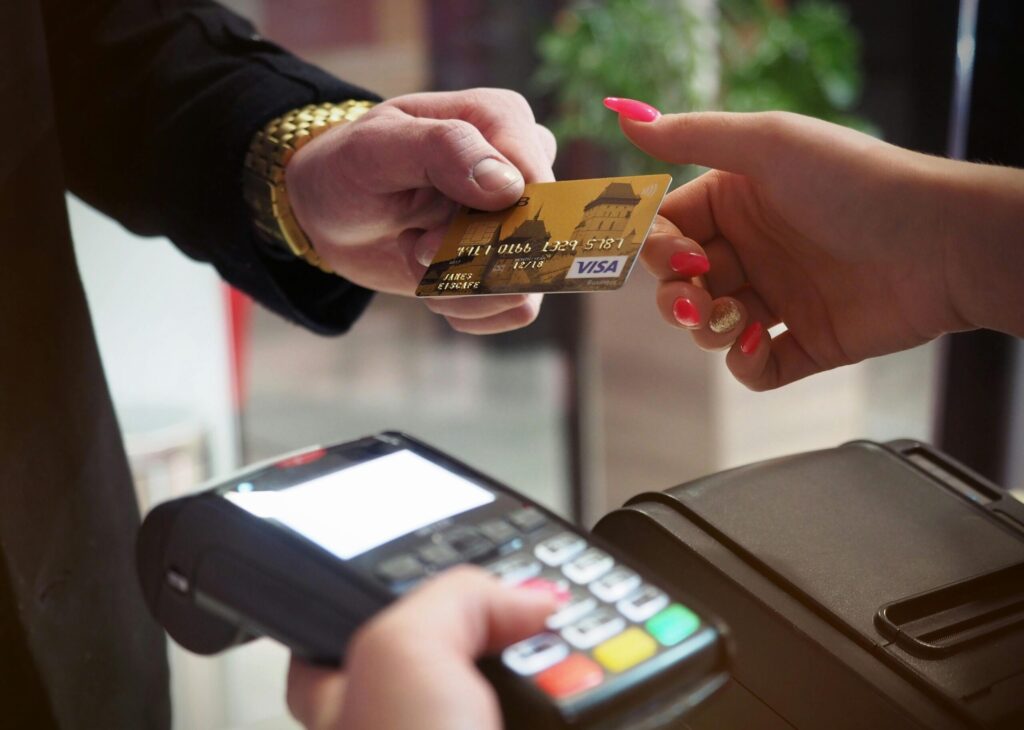
The Evolution of Credit Analysis
Traditionally, credit analysis involved manual processes that required intensive labor and were prone to human error. Analysts would sift through financial data, employment records, and credit histories, applying predefined criteria to assess risks and approve loans. While these methods offered personalized assessments, they lacked scalability, precision, and the ability to adapt dynamically to market changes.
With the advent of financial technology and the increasing reliance on data analytics, automation has emerged as a game-changer. By incorporating machine learning algorithms and AI-powered platforms, institutions can now process vast amounts of data in real time, making credit analysis faster, more reliable, and highly scalable.
Key Advantages of Credit Analysis Automation
Enhanced Efficiency and Speed
Automated credit systems significantly reduce the time needed to evaluate creditworthiness. Through machine learning models, institutions can analyze massive datasets almost instantaneously, accelerating the decision-making process and improving the customer experience.
Improved Accuracy and Reduced Bias
Human biases and errors are common pitfalls in manual credit analysis. Automation minimizes these risks by applying consistent evaluation standards across all applications. Algorithms can objectively assess credit histories, current financial situations, and market trends to produce highly accurate risk assessments.
Better Risk Management
Risk management is a critical aspect of financial operations. Automation tools can predict potential defaults more effectively by analyzing patterns that humans might overlook. Early detection of red flags allows financial institutions to mitigate risks proactively and strengthen their portfolios.
Cost Savings
Reducing the need for manual labor lowers operational costs. Automation also enables the reallocation of human resources to higher-value tasks, such as strategy development and customer relationship management.
Enhanced Compliance
With evolving regulations, financial institutions must ensure compliance to avoid penalties. Automated systems simplify compliance monitoring by continuously analyzing data and flagging anomalies, thereby reducing legal and reputational risks.
Core Technologies Driving Automation in Credit Analysis
Artificial Intelligence (AI)
AI forms the backbone of credit analysis automation. By simulating human intelligence, AI systems can interpret complex data patterns, draw insights, and make decisions with minimal human intervention. AI’s adaptability ensures that models evolve alongside changing financial environments.
Machine Learning (ML)
Machine learning is crucial for enhancing the predictive accuracy of automated credit systems. ML algorithms are designed to learn from historical data and improve over time, providing refined credit scoring and fraud detection capabilities.
Big Data Analytics
Big data platforms enable financial institutions to leverage enormous volumes of structured and unstructured data. Real-time data processing ensures that credit analysis is based on the most up-to-date information, enhancing the reliability of assessments.
Natural Language Processing (NLP)
NLP facilitates the analysis of unstructured text data, such as loan applications and financial reports. By interpreting qualitative data, NLP adds another dimension to automated credit systems, providing a comprehensive view of an applicant’s creditworthiness.
Implementation Strategies for Financial Institutions
Invest in Robust Technology Platforms
Adopting reliable automation platforms is critical. Financial institutions must partner with technology providers who offer scalable and secure solutions tailored to their needs.
Focus on Data Quality
Automation relies heavily on data quality. Institutions must ensure that their datasets are accurate, comprehensive, and regularly updated. This includes integrating data from various sources, such as credit bureaus, bank statements, and tax records.
Employee Training and Change Management
Transitioning to automated systems requires a shift in organizational culture. Employees need training to effectively use new tools and interpret automated insights, bridging the gap between technology and human expertise.
Monitor and Refine Models Continuously
Automated systems require regular monitoring to ensure optimal performance. Institutions should periodically refine their algorithms, considering feedback and new market data to maintain accuracy and relevance.
Challenges in Adopting Credit Analysis Automation
Despite its numerous benefits, automation is not without challenges. Data privacy and cybersecurity remain significant concerns, as the integration of vast datasets increases exposure to potential breaches. Additionally, the initial investment in automation technology can be substantial, posing a hurdle for smaller institutions.
Regulatory hurdles also present challenges. Compliance with diverse and often stringent financial regulations can complicate the deployment of automated credit systems. Therefore, it is essential for institutions to work closely with regulators to navigate this landscape effectively.
The Future of Credit Analysis Automation
The future of credit analysis lies in further innovation. Advances in blockchain technology may soon enable more secure and transparent credit assessment processes, while the integration of predictive analytics could make risk assessments even more precise. Additionally, open banking initiatives are likely to enhance collaboration between institutions, providing a broader and more holistic view of customer data.
As customer expectations evolve, financial institutions must embrace continuous innovation. Personalization through AI-driven insights will become a key differentiator, enabling lenders to offer tailored credit solutions that meet individual needs.
Conclusion
Credit analysis automation is undeniably transforming the financial industry. By harnessing the power of AI, machine learning, and data analytics, institutions can streamline their operations, enhance risk management, and deliver unparalleled value to customers. However, success in this domain hinges on addressing challenges such as data privacy, regulatory compliance, and initial implementation costs. With the right strategies and technologies, financial institutions can unlock the full potential of automated credit systems and stay ahead in an increasingly competitive market.