Mathematical models in finance play a pivotal role in driving modern economies. Their ability to forecast trends, mitigate risks, and optimize decision-making processes has made them indispensable. From predicting market fluctuations to managing risks effectively, these models are designed to interpret complex data sets, uncover hidden patterns, and provide actionable insights.
This article explores how these models have revolutionized finance, the principles behind their success, and their real-world applications. Understanding these systems can empower businesses and individuals alike to navigate the intricacies of financial markets effectively.
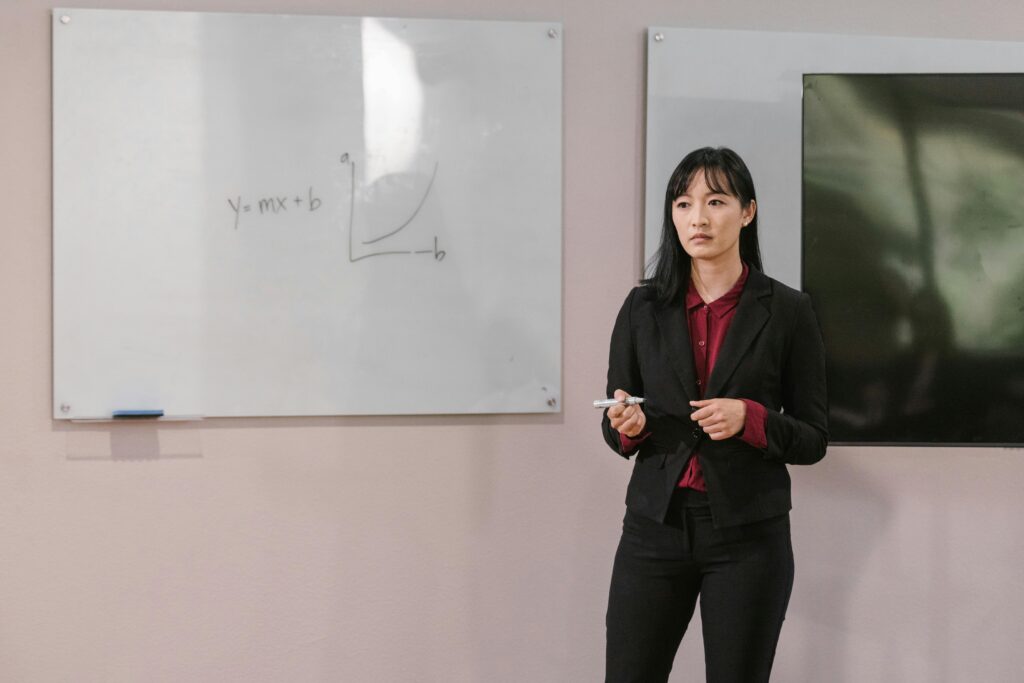
The Importance of Mathematical Models in Finance
Quantifying Complexity
Financial markets are intricate, with numerous variables affecting outcomes. By utilizing mathematical models, financial analysts can quantify complexities and make well-informed decisions. These models can:
- Predict stock prices.
- Manage portfolio risks.
- Simulate economic conditions under various scenarios.
Mathematical models serve as tools for turning uncertainty into actionable insights, enabling decision-makers to strategize effectively even in volatile markets.
Bridging Theory and Practice
Modern mathematical models embody theoretical knowledge and translate it into practical applications. For example, concepts like the Black-Scholes model use partial differential equations to price options. Such methods are grounded in academic rigor but directly influence trading floors worldwide.
Key Characteristics of Successful Financial Models
1. Accuracy in Forecasting
A model’s efficacy largely depends on its ability to provide accurate forecasts. Predictive algorithms incorporating machine learning often outperform traditional models by continuously adapting to new data. These adaptive systems are particularly vital in:
- Credit risk assessment.
- Currency exchange predictions.
- Pricing derivatives.
2. Robustness
Robust models can perform well under different market conditions. They incorporate stress-testing mechanisms to ensure resilience during financial crises. Robustness is crucial for long-term sustainability and helps organizations withstand economic shocks.
3. Scalability
Financial markets are dynamic, requiring models that scale effectively. Scalability ensures that models handle increasing complexities as markets grow. Scalable systems are designed to manage larger data volumes, multiple asset classes, and diverse economic conditions seamlessly.
Popular Mathematical Models Used in Finance
1. Black-Scholes Model
Developed by Fischer Black and Myron Scholes, this model is a cornerstone of modern finance. It evaluates the price of options, offering insights into:
- Expected volatility.
- Fair market value of derivatives.
Its widespread use underscores its relevance and enduring impact on global trading practices.
2. Value at Risk (VaR)
The Value at Risk model calculates the potential loss of an investment portfolio over a defined time frame. It enables companies to:
- Assess exposure to financial risks.
- Optimize risk mitigation strategies.
- Demonstrate regulatory compliance.
3. Monte Carlo Simulation
Monte Carlo methods use randomness to solve problems that might be deterministic in nature. They are extensively applied in:
- Forecasting stock price movements.
- Analyzing the likelihood of investment outcomes.
- Derivative pricing scenarios.
4. Capital Asset Pricing Model (CAPM)
CAPM evaluates expected returns based on systematic risks compared to market benchmarks. It has significant applications in:
- Portfolio optimization.
- Understanding the cost of capital.
- Benchmarking fund performance.
Predictive Analytics and Machine Learning
Transforming Data Into Insights
Predictive analytics, enhanced by machine learning, has revolutionized the accuracy and application of financial models. Neural networks and decision trees enable systems to:
- Identify market trends.
- Detect anomalies in large data sets.
- Offer real-time insights to traders.
Automation in Algorithmic Trading
Machine learning underpins many algorithmic trading systems. These models execute trades based on predefined rules and real-time data, delivering:
- Reduced manual intervention.
- Faster transaction times.
- Increased profit margins through efficient pricing strategies.
Mathematical Models and Risk Management
Mitigating Financial Risks
Accurate models improve risk management frameworks, identifying vulnerabilities before they escalate into significant losses. Approaches include:
- Stress testing under extreme conditions.
- Scenario analysis to evaluate potential outcomes.
- Credit scoring techniques to assess borrower reliability.
Behavioral Insights
Financial models also integrate behavioral economics, understanding that human emotions like fear and greed significantly affect markets. By modeling these variables, systems can better predict sudden shifts.
Ethical and Regulatory Considerations
Transparency and Accountability
Despite their efficacy, financial models are not without challenges. The 2008 global financial crisis highlighted how over-reliance on complex models without transparent mechanisms could exacerbate problems. Moving forward, ensuring accountability in model applications remains a priority.
Balancing Innovation with Compliance
As financial models evolve, they must align with ever-changing regulations. Balancing innovation with strict regulatory frameworks is vital for maintaining trust and market stability.
Conclusion
Successful mathematical models in finance epitomize the blend of innovation and practicality. They continue to reshape the financial landscape by enhancing risk management, forecasting accuracy, and operational efficiencies. However, as these models grow more sophisticated, their ethical implications and regulatory compliance cannot be ignored.
By embracing the power of these systems while addressing their limitations, the finance industry can drive sustainable growth, navigate uncertainties, and harness opportunities for long-term success. Ultimately, mathematical models remain not just tools but pivotal enablers of a more informed and resilient global economy.